1. July 2024 By Milena Fluck and Andy Schmidt
Decision Making under Uncertainty
When we make decisions – no matter how important they are – it does not only matter who makes the decision, but also her or his surroundings. We define risk as the potential that a decision will lead to loss or an undesirable outcome. Whereby risk is the multiplication product of consequence and probability. As for roulette in the European variant within 30 rounds you have a 45% chance (probability) of getting the number never right at all (consequence). This is a perfectly defined risk of losing. In the real world it is often not as easy to define. Therefore, we need to distinguish between decisions made under risk and uncertainty about those risks. How probable is the risk, but also are we certain about all risks that exist?
Information is the resolution of uncertainty.
Claude Shannon
This kind of uncertainty is referred to as epistemic or systematic uncertainty and means the incomplete state of scientific knowledge. This form of uncertainty can be reduced by additional information. Often, we acquire more information by hiring experts and consultants. Even though new information can decrease uncertainty, it can also increase uncertainty, as it may reveal the presence of risks or unknown probabilities of risks that were previously unknown or were underestimated.
Aleatoric or statistical uncertainty on the other hand refers to the notion of randomness. There is a randomness in the world we just cannot predict. Black swan events describe unpredictable events that are beyond what we would normally expect and that have potentially severe consequences. For a long time, people only believed there were white swans until black swans were discovered in Australia when no one expected it. Events considered black swan events are among others the 2007 financial crisis and the nuclear disaster in Fukushima. Think of black swan events in your projects. Things you could have never seen coming even though you might wonder afterwards.
When we know all the alternatives and their probabilities and consequences regarding a decision, we have a manageable small world as our solution space in which we can optimize the decision-making process and the decision. Not in an uncertain world though. We deal with risk versus uncertainty, a distinction that is of fundamental importance. Optimization methods such as Bayesian inference provide the best possible answers. But not in the large uncertain world, where information is unknown or unknowable. Whereas most decisions in the real world are made under uncertainty. The real world is a non-graspable system with way more complexity and dependencies. Decisions in this kind of world range from “Should I order food tonight?” to “Who should I date?”.
Eventually we might be able to figure out all risks and their probabilities and we will find the optimal solution via computation, but daily we make such decisions with much less consideration. Some calculations would take a lifetime and then of course we have black swan events. We might never be able to find a perfect solution for some decisions – whether we are human or a machine. This is why we are using different cognitive processes in both worlds. While in a world with fully known risks and alternatives we can use statistical thinking and computationally track our gains and losses, which gives us the option to adjust. In an uncertain world we often have to rely on simple and fast heuristic thinking. But how does making decisions under uncertainty work exactly?
There are three main strands of research we are going to look:
- 1. In normative approaches research has examined how people should make decisions.
- 2. Descriptive approaches on the other hand research how people make decisions already.
- 3. The third and descriptive field of research are heuristics which gained a lot more interest during the last decades.
The Decision Maker
Homo Oeconomicus
In economic theory the Homo Oeconomicus is used as a model of an ideal person who thinks and acts exclusively according to economic criteria. He acts rational and strives for the greatest possible benefit. Further, she has complete knowledge of her economic decision-making, consequences and complete information about markets and goods.
Still mathematical economics is aware there is a certain subjective influence even for rational agents like the Homo Oeconomicus. The expected utility theory therefore presupposes that rational agents should maximize utility – the subjective desirability of their actions. Utility is subjectively relative. For a homeless person one euro can secure a dinner, while a multimillionaire might lose a euro without ever noticing it. It remains the same coin but represents a completely different measure of utility. The marginal utility decreases with every euro we gain.
One famous example: Imagine you find a lottery ticket. With a probability of 50 % you win 1 million euros, while you have the same chances of getting nothing. Now a multimillionaire comes along and offers 500.000 euros to buy the ticket from you. Will you keep or sell it?
Normative approaches like the expected utility theory now try to guide the Homo Oeconomicus on how to make the best decision in this case. Decision making based on the expected utility theory requires one to assess the probability of all relevant system outcomes. The value of every additional euro gets lower the more you have.
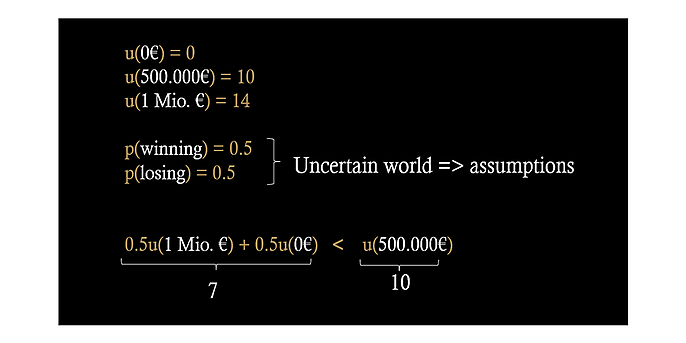
Going from having nothing to gaining half a million is evaluated higher in utility, than going from half a million to one million. Therefore, utility for 500.000 euros might be at ten, while you might consider the million only at 14. In this case the probabilities of winning and losing are clear. In an uncertain world we will have to make assumptions on the probabilities and determine the subjective utility. Summing up the probability times the subjective utility for all given outcomes gives us the results for overall utility for each scenario. If we compare these, we get our answer, which might differ depending on the reader’s assets.
Typically, the utility function is almost linear within a range that is small compared to the working capital of the decision maker. For a large insurance company, the curve might appear linear in a data section where a small engineering consultancy already feels the effects of marginal utility. For a huge insurance company collecting millions of euros every year making “small” decisions is not as severe as for a small engineering company with only a couple thousand euros. This “size effect” is an underlying business model of insurance companies. The insurance company can take on the risks with known probabilities, risks with unknown probabilities and unpredicted occurrences of events with negative financial consequences for their clients, who are themselves unable to cope with these, in a world of uncertainty.
In the real world we of course must consider other factors than money and their utility. E.g. the utility of employees staying healthy or a decision's impact on climate change. From an ethics point of view therefore we most wonder from whose perspective utility is determined. And can factors such as the health of employees be weighted economically like money? This becomes important in cost effective policies. The aim of a policy is to achieve a policy objective at lower cost than other policies. Cost and effectiveness should be evaluated from a societal perspective. Utilitarianism is the sum of all individual utilities, while cost and benefit then can be measured in expected utility units. This is why in New Zealand fences in swimming pools prevent kids from drowning, but there are no shark nets installed for the rare event of a shark attack even though the death is equally tragic. Installing fences in a swimming pools is less expensive while saving more lives. It therefore is considered to be more cost-effective.
Humans
In contrast to Homo Oeconomicus humans most of the time are not perfectly rational, but also, they are not entirely economically motivated. Humans are individuals with cognitive limitations and affective biases. This is why there might not be a recipe to tell people how to make optimal decisions and why another strand in research is to explore how people actually make decisions under uncertainty. We will now look at descriptive approaches.
One important theory in this area is the prospect theory, a theory of behavioral economics developed by Daniel Kahneman and Amos Tversky in 1979. The probabilities of occurrence of possible environmental influences are not weighted objectively but using a probability weighting function. The benefit for the decision-maker is not measured based on the absolute benefit. It refers to a reference point and the change to this reference point. As an example: a glass of water can be worth a few pennies if you are sitting at the office and are not thirsty and it can be worth a lot if you are alone in the desert, close to dying of thirst. Losses are weighted more heavily than gains due to various possible explanations. In the area of losses, the behavior of individuals is also much more risk-taking than in gains. As a result, the occurrence of improbable events is often evaluated as too high in the area of loss.
The pain of losing something is psychologically a lot more powerful than the pleasure of gaining. One explanation is the endowment effect. It states that things have a higher value that are of symbolic or emotional significance for us. Imagine you got an old and ugly car when you turned 18. You now got a new, fancier car. You now sell your old rattletrap and will still expect a lot more money for it that you would be ready to pay when you were to acquire it yourself. Psychological ownership creates an association between you and the item which leads you to increased perceived value of the item. The endowment effect might also explain why studies find higher rates in risk taking demeanor in professional decisions – so on behalf of the corporation – compared to risk taking behavior in private decisions.
Another human anomaly in decision making is the status quo bias. People have the tendency to do nothing when facing a decision. We rather stick with the norm as long as the default option is viewed positively. Why pick a new dish from your go-to restaurants menu if you can be sure the one meal you always chose will not disappoint you. This can also be seen in economics where there is no change in investment even though there are no returns.
Why?
- First, we have a misconception of adhering to the default option as “the safe thing” and are scared to regret any changes later. Referred to as regret avoidance.
- Second, sticking with the status quo helps us to avoid deciding at all, which we do not really like making decisions since they cause stress. Keep in mind that keeping the status quo is especially attractive for people that are satisfied with their status. Referring to the example above these are the people in the office and not the desert.
- Further, not deciding at all and sticking with the default can also be considered a decision.
Making decisions whether we make them for us or a whole society come with consequences. These can either be monetary or non-monetary, such as impacts on comfort and convenience. Consequences in time as “I will be late”, social life, career or health.
On the other hand, by deciding we might receive rewards. We have primary rewards, such as food, safety and water, that concern our survival and simply are biological needs, as well as secondary rewards that can be used to access further primary or secondary rewards. With money you can buy a security system for your house and therefore buy more safety.
Humans understand the relationship between risk – consequence multiplied by its probability – and reward. The risk reward heuristic asserts that we link high payoffs to low probabilities in receiving a reward and the reversed way.
Imagine in a raffle one can win a PlayStation 5. We will assume it is unlikely we are going to win. While if one can only win a chocolate bar, we might assume our probability of winning to be much higher. Even if we do not know the chances of winning the raffle. It might be the same chance to win in both cases. People estimate missing probabilities from learned risk-reward-relationships.
Further impacting factors are reversibility and revocability of consequences. Reversibility describes the possibility of returning to a previous state. Impacts of reversibility are governed by physical laws. Amputations of body parts or the melting of Greenland ice sheets simply cannot be reversed. In economics reversibility is rather referred to as revocable. Revocability describes the duration and cost it will take to revoke a decision. As an example, one decides to sleep longer. The consequence is missing a booked plane. How much will it cost to get a new ticket? How much later will one arrive?
Reversibility is often discussed regarding climate matters. When talking about natural resources we often must consider extreme revoking costs, long-term effects and the uniqueness of these resources. If we consume all fossil fuels, are we going to be able to substitute them?
In economics irreversibility does generate a reluctance to invest. This means that the sales price is lower than the purchase price. This generates loss. If the purchase price cannot be recovered by generated revenue in the future revoking costs for the decision to purchase are too high and the decision becomes irreversible.
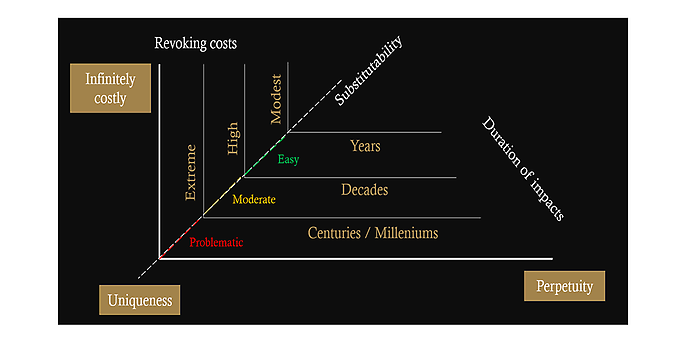
Let us look at another human factor. To become federal chancellor, you have to have a minimum age of 18, for federal president 40 and to become US president 35. But does age really help you to make more societal favorable decisions under uncertainty. Imagine you got a speeding ticket. Next time you go fast you might remember that unpleasant feeling and eventually slow down to prevent getting another ticket. You remember that feeling – a somatic marker has been set. Somatic markers are changes in the body, such as heart racing, anxiety or heat, that are associated with emotions.
According to the somatic marker hypothesis, somatic markers strongly influence subsequent decision-making. This means we learn from mistakes because we can reactivate these emotional states. And even better, one can use these somatic markers in an as-if-body-loop: we can just imagine getting another speeding ticket and imaginarily reproduce that unpleasant feeling. It might not be the age, but people with more experience in life could be more likely to be successful with a “follow your gut”- strategy.
Ecological Rationality
Humans cannot optimize decisions by statistical calculations not only due to limited resources, but also because they are humans in different environments and with individual perspectives. Time for deciding is always limited. Collective decisions may take longer than individual decisions. Further, we have limited available information and a subjective perception of the world. We are not perfectly rational. Instead, we adapt our decision strategies to different environments as suggested by the concept of ecological rationality, another descriptive approach in decision making.
Imagine you want to cross a big intersection with traffic lights. The rational behavior would be: Go when you see a green mannequin, stay when you see the red one. If all participants in road traffic stick with that basic logic no one should ever get hurt unless traffic lights are broken.
You are in a hurry though, so you do not want to wait for the lights to turn green. This is not an immediate reaction but still a process of careful consideration, that has some time pressure attached to it. Every second that goes by not having decided will reduce the expected reward of saving time and you will have to reconsider. You might consider whether you can hear the lights and sound of an arriving vehicle. But on the other hand, it is raining bad, so you might not see the lights. Also, you have read about electric cars not making any sounds when driving. But then again there are not many of these cars yet on the streets. Also, it is two o ‘clock in the morning. The chances of vehicles coming is low. You decide to cross the street under various circumstances within seconds, and it might not always be optimal to do so. That is one reason why we have traffic accidents in intersections.
In rationality decisions are based on or in accordance with reason and logic. Bounded rationality is a process in which we attempt to make a decision that is satisfactory rather than optimal. We are talking about decisions that are made under consideration, but due to uncertainty we do not know all probabilities and facts to consider. Further, there are just too many factors we could consider. This is where the adaptive toolbox comes in. A collection of fast-and-frugal heuristics. With heuristics we ignore part of the information in the decision process. Heuristics are ecologically rational because decision making using them is effective under circumstances of bounded rationality. We make decisions more quickly and frugally than with more complex methods. Still often optimization theories are perceived to be more accurate when it comes to making “optimal” decisions. Erroneous beliefs in this area are:
- 1. Heuristics produce second-best results in decision making; optimization is always better.
- 2. We only use heuristics due to limited cognitive resources.
- 3. People rely on heuristics only in routine decisions of little importance.
Let us look at one heuristic important in decision making. Of course there are many more.
The 1/N heuristic or equality heuristic
This heuristic leads to allocating all resources equally to each of N alternatives – so all the options. One example for parents is to distribute time and attention on all kids equally. In many situations, fairness and justice are achieved by distributing resources equally.
A financial study investigated 14 portfolio rules.
These optimization rules are all about how to weight assets in a portfolio. Among these rules was the sample mean variance and multiple Bayesian approaches. There were seven empirical datasets used. Among others the MSCI. All with data from the last 21-41 years. With 1/N naive diversification all assets are weighted equal, referred to as an equally weighted portfolio. This achieved better out-of-sample prediction than any of the optimization models in terms of Sharpe ratio, certainty equivalent and turnover. While optimization models eventually achieve better results after more time of estimation. To outperform 1/N for a portfolio with 25 assets the estimation window is more than 3.000 months with parameters calibrated to the US Stock-market. This shows how a simple heuristic outperforms optimization models for big markets with great uncertainty disproving belief number one.
So, heuristics work better in a world of uncertainty and outperform optimization strategies. But why? One explanation is the bias-variance-dilemma. We want our models to predict the future accurately, but this takes effort. This leads to the accuracy-effort-tradeoff. With optimization we continually minimize prediction-errors. The more we compute the better our precision. But this only works if we can measure our performance. Are we getting better at predicting the outcomes of a decision? This is hard to measure in a world of uncertainty. Heuristics on the other hand use a lot less information and computation. They often make decisions based on one reason, fast and frugal ignoring further cues.
Why would using less information and computation lead to more accuracy? For deciding we should investigate data we have from the past. What do we know already? We then must use fitting to detect patterns that might guide us to the second step of prediction. While fitting is of course important, prediction is the really important step, because whether we make a good prediction might conclude whether we survive. Humans forget, machines do not. Therefore, they have a lot more data to use for fitting. In a lot of data, you can find a lot of outliers. In statistics these outliers create variance in the data and lead to an error in fitting based on sensitivity to minor fluctuations in the test data. This is called overfitting.
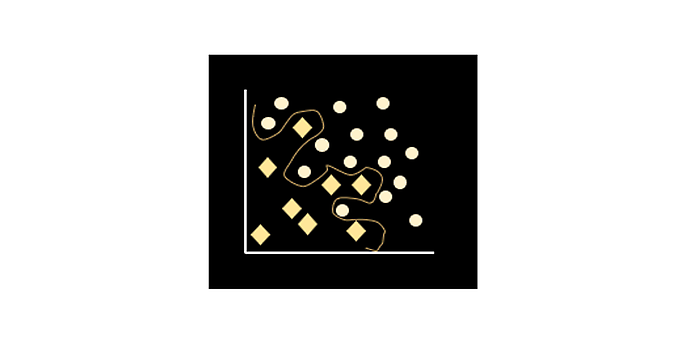
We can now adjust our data by learning about the outliers and simplify it by straightening the line and finding a good compromise. Wrong assumptions in learning algorithms though might lead to underfitting and oversimplification.
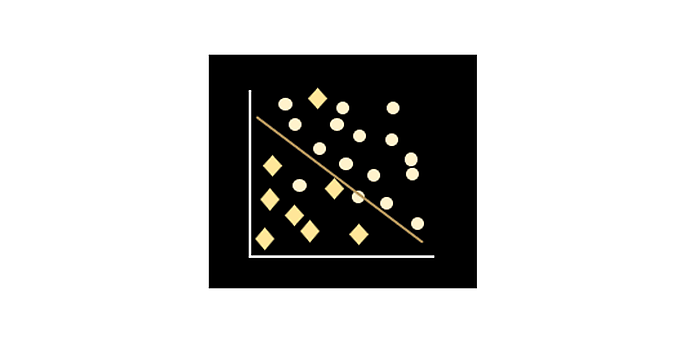
The bias-variance dilemma by the way still is a problem in supervised learning, such as classification and regression. Even though it could be shown that large language models from Open AI are already applying some kinds of heuristics.
How do we deal with Uncertainty in IT?
In IT Workflow
As we all know projects face unexpected changes on the market, in the IT industry, the organization or in law. A couple decades ago some people concluded that when using waterfall models in IT development decisions had to be made too early in the process. What if the initial decision has not proven to be satisfying? What about unforeseen changes on the market? There was a need for iterative, incremental, evolutionary models where decisions can be made when the time is right. In the 1970s there were first approaches with evolutionary management and adaptive software development.
Postponing decisions, decomposition and therefore slowly gaining information as well as keeping reversibility in mind are fundamental requirements in agile frameworks. It helps us to postpone decisions until we can safely make them and reverse decisions that lead to negative consequences fast and cheap. As the Agile Manifesto tells us: reacting to change is more important than following a plan.
In Software Architecture
Architects are often confronted with major decisions under uncertain circumstances. Within architecture there have been some proposed approaches on how an architect can deal with decisions under uncertainty. The area of architecture is aware of epistemic uncertainty. As Neal Ford and Mark Richards show with this pyramid.
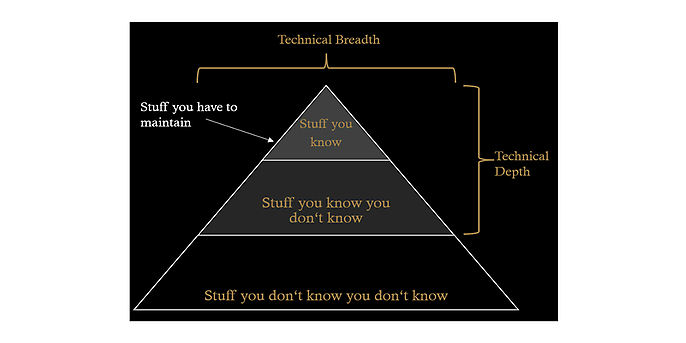
There is deep technical knowledge on programming languages and frameworks that needs to be maintained. Further there is the stuff an architect knows he does not know. While there are also the things architects do not know they do not know. This area of the pyramid should preferably be minimized. If possible, architects are often advised to postpone a decision and keep as many options open as long as they can. Sometimes there is no way around deciding immediately. In that case it is important to keep revocability in mind. A single and perfectly decoupled micro service in example can later be replaced much cheaper and faster than tiny pebble stones in a big ball of mud.
A good architect maximizes the number of decisions not made.
Robert C. Martin, Clean Architecture
In Management
In management oftentimes it is assumed that all reasons for a decision need to be justified by numbers. In a world of uncertainty, we do not have all the numbers. In 2006 twelve Swedish managers in the forestry industry were asked how they dealt with risky decisions. Top answers were “delegate the decision”, “avoid taking risks'' and “delay the decision”. Further measures they mentioned were “carrying out a pilot-study” or “sign-away at least part of the risk to partners”.
In a big study in 2012 with 1.000 US CEOs, the managers were asked to rank factors that influenced their decisions on capital allocation. So, how financial resources are allocated to projects, which is a major and recurring decision in organizations. From a total of ten possible answers “gut feel” was ranked at place seven.
In Organizations
Everybody makes decisions within an organization. Whether the organization is a sports club or a big corporation. Decisions are either made centralized or decentralized. Further, depending on the existing culture they are made autocratic or democratic, most likely when there is a high level of trust in subordinates. Often some kind of built-in culture guides people on what is right and what is wrong.
Conclusion
There is uncertainty and it can be important to be aware of the unknowns and to accept them. There is no general instruction on how to make the optimal or rather most satisfying decision.
We demand rigidly defined areas of doubt and uncertainty!
Douglas Adams, The Hitchhiker’s Guide to the Galaxy
In our daily work life, we constantly have to make small and big decisions under uncertain circumstances. Humans have a great embedded toolbox on how to deal with these. Additionally, some strategies that have already proven to be helpful when coping with decisions made under uncertainty in IT are:
- 1. Postpone decisions if possible and minimize uncertainty.
- 2. Disassemble problem domains in challenges with complete certainty.
- 3. Keep it (systems, solutions, documents etc.) evolutionary.
- 4. It is ok to use heuristics (smart).
You can find out more about exciting topics from the world of adesso in our previous blog posts.