30. January 2024 By Tim Bunkus
What is generative AI?
Generative AI has been the talk of the town ever since ChatGPT was launched. But what is it and how is it different from machine or deep learning? In this blog post, I explore the many aspects of AI.
Artificial intelligence – all the rage since 1956
The term ‘AI’ was first coined 1956 by the computer scientist John McCarthy, who described it as ‘the science and technology of making intelligent machines’. Since then, it has gone on to become an interdisciplinary field of science that focuses on simulating, understanding and improving the intelligent behaviour of machines.
The origins of AI can be traced back to the early days of computer science and mathematics. Way back in the 17th century, the philosopher and mathematician Gottfried Wilhelm Leibniz developed a universal language of logic, which he called characteristica universalis. He dreamt of a machine that could draw logical conclusions and generate knowledge. Let us now fast forward to the 20th century when the foundations of modern AI were laid in the work of Alan Turing, Claude Shannon, Norbert Wiener and others who focussed on computability, information theory, cybernetics and artificial neural networks.
To make the expansive field of artificial intelligence easier to understand, we draw a distinction today between three major AI methods: machine learning, deep learning and generative AI. These developed one after the other and build on each other. Each of them will be described in greater detail below.
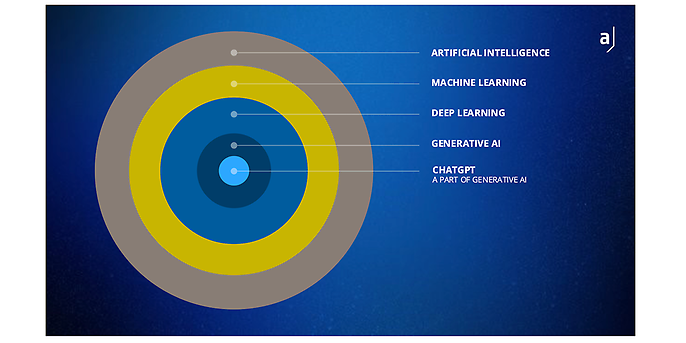
Machine learning – simple, learning algorithms
Machine learning (ML) is a field of AI that deals with the development of algorithms and models that can learn from data without being explicitly programmed. ML is the driving force behind the first wave of AI, which began in the 1980s and was primarily based on statistical methods. ML is underpinned by statistics, as they are what make it possible to identify patterns and correlations in large amounts of data and make predictions.
Explainability of ML models and the advantages this bring
One advantage of ML models is that they can be explained in many cases. In other words, it is possible to see how they arrived at a particular decision or recommendation. This is important if you want to increase trust and acceptance amongst users, provide accountability and transparency, and correct for potential errors or biases. Explainability is critical in applications with high ethical or legal standards, such as in medicine, in finance or in the public sector.
Applications for ML at the moment
Today, ML is used in a variety of areas to solve or optimise complex problems. Here are a few examples:
- Recommendation systems that allow you to generate personalised recommendations for products, services or content
- Anomaly detection, which makes it possible to spot unusual or suspicious patterns or activities in data
- Predictive analytics that lets you predict future events or trends on the basis of historical data
Deep learning – complex learning algorithms
Deep learning (DL) is another field of AI that deals with the design of artificial neural networks that consist of several layers of interconnected artificial neurons. It is the driving force behind the second wave of AI, which got started in the 2010s and was made possible mainly due to the availability of vast amounts of data and compute. Artificial neural networks are replicas of human thought processes that can be used to model and learn complex non-linear functions.
Loss of explainability – scalable complexity comes at a cost
One advantage of DL is its scalable complexity, which can be defined as the ability to extract and visualise increasingly complex and abstract features from data. This leads to improved accuracy and performance in many applications, especially those involving unstructured data such as texts, images or audio. One drawback of DL, however, is the loss of explainability, this being the case because it is difficult from a mathematical perspective to understand how artificial neural networks arrived at a particular decision or recommendation. This could lead to a lack of trust, transparency and accountability, not to mention potential errors or biases.
Current areas of application for deep learning
Today, DL is used in a variety of areas to open up new opportunities or improve existing solutions. Here are a few examples:
- Computer vision, which makes it possible to process and understand visual information
- Natural language processing
Generative AI – complex, information-generating algorithms
Generative AI (GenAI) is another subfield of AI that deals with the development of models that are able to generate new data or content similar to the information they have been trained on. GenAI is the driving force behind the third wave of AI, which began in the 2020s and is chiefly based on the development of transformer models.
Transformers are coming
The transformer architecture is a novel type of artificial neural network that was presented back in 2017 in a paper titled ‘Attention is all you need’. The architecture is based on the concept of attention, which allows the most relevant parts of an input to be identified and weighted to produce an output. The transformer architecture consists of two main components: the encoder and the decoder. The encoder converts the input into a series of vectors that contain the semantic and syntactic information of the input. The decoder generates the output based on the vectors of the encoder by paying
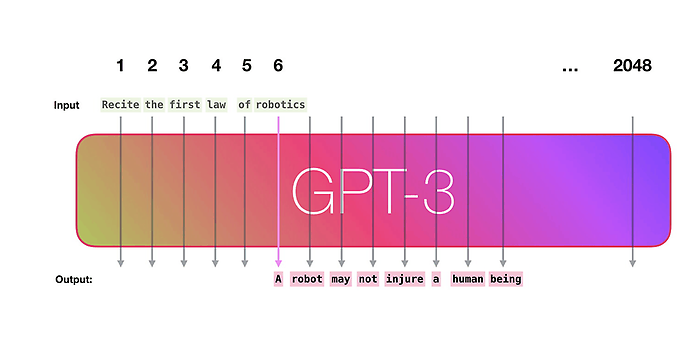
Vectors – mathematical sorting tools
What all machine and deep learning methods have in common is that the models ultimately perform mathematical operations. And because these operations are based on numbers, any unstructured information such as texts, images or audio data must first be converted into numbers. Each unit of information, be that a word, a pixel or an amplitude, is assigned a vector that contains the properties of this unit. By using vectors, the information can be presented in a common (mathematical) space in which the similarity or relationship between the information can be measured based on the distance or angle between the vectors.
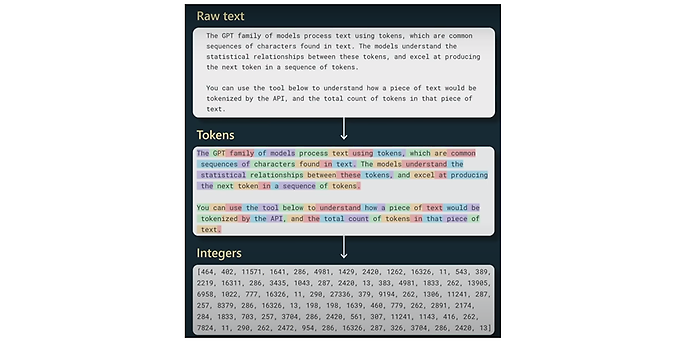
Vectors allow you to visually render text information and other types of information such as audio data, images or even proteins. This opens up new possibilities for GenAI to generate or process different types of information that are not based on natural language. Here are a few examples:
- Jukebox: a model that is capable of generating music by being pre-trained on a large corpus of music and then applying this knowledge to carry out various tasks such as creating a particular style of music, writing lyrics or composing music.
- CLIP (Contrastive Language–Image Pre-training): a model that is able to understand images by being pre-trained on a large corpus of images and texts and then applying this knowledge to perform various tasks such as image classification, image searches or image labelling.
- AlphaFold: a model that is capable of predicting the three-dimensional structure of proteins by being pre-trained on a large corpus of proteins and then applying the knowledge to carry out various tasks such as protein design, interaction or function.
Multimodal models – the true all-rounders
Multimodal models are GenAI models that are able to generate or process several types of information at once, such as text and image data, text and audio data or image and audio data. This requires a high degree of complexity and integration as this relates to the models, seeing as they have to be able to combine and coordinate the different bits of information to produce a coherent, logical output.
Use cases for generative AI
There are a wide range of possible applications for generative AI. It can carry out any task it is called on to perform, in combination with standard machine and deep learning, semantic searches and knowledge databases. For clarity’s sake, we distinguish between three types of application areas:
Domain knowledge agents
A domain knowledge agent is a GenAI model that is capable of generating or providing information by being pre-trained in a specific domain and then applying the knowledge to carry out various tasks such as knowledge transfer, knowledge testing or knowledge generation.
GenAI in application development
GenAI in application development involves the use of GenAI models to support or speed up the development of applications by creating or enhancing various parts of the application such as its design, functionality or contents.
Copiloting in text and image production
Copiloting in text and image production involves the use of GenAI models to support or improve the production of text or image content by generating or optimising various aspects of the content such as its quality, the level of creativity or its relevance.
Outlook and conclusion
Generative AI is an exciting, innovative field of science that is producing more and more models with special capabilities that can generate or process different types of information. These models offer new ways to interact, collaborate and be creative, which is why they have the potential to integrate and revolutionise all communications. However, this does not mean that the ‘traditional’ methods of machine and deep learning are no longer needed. They are still well suited for carrying out special tasks or meeting requirements that demand a higher level of explainability, robustness or efficiency.
If we think back to Gottfried Wilhelm Leibniz and his dream of a machine that generates knowledge and is able to make logical inferences, it is clear to see that each new iteration of AI methods is bringing us one step closer to making this dream a reality. GenAI is not yet able to demonstrate universal, or general, intelligence that can be applied to every situation or problem. Even so, it does allow us to address a variety of company-specific challenges through the (humanly) intelligent combination of different AI methods.
Would you like to find out more about GenAI and what we can do to support you? Then check out our website. We offer you a concise overview of all topics relating to GenAI with an array of podcasts, blog posts, events, studies and much more.