24. February 2023 By Nadine Lamprecht
Vision of the future – the self-driving supply chain
As a manufacturing company, we see our suppliers’ stock levels in the system and our Customer Service department can say exactly when the delivery will be made. Bottlenecks no longer exist because the needed quantities have been (re)ordered with the help of predictive monitoring in order to get them to the customer as quickly as possible. Would that not be wonderful? And in the future, it will actually be possible.
Several steps have to be prepared for this centralised system, which has access to all the relevant information and is equipped with analysis and decision-making algorithms, such as machine learning. And the milestones along the way already reduce the number of manual operations needed and thus save time and money.
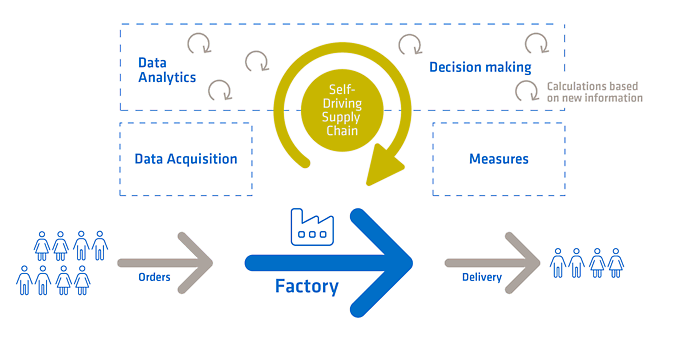
Data collection
Central data collection forms the basis for the self-driven supply chain. This means that the systems companies own (including ERP, WMS, TMS, CRM or APS) are connected with interfaces. Manual sub-processes need to be eliminated as well – for example, with the help of robotic process automation.
And it is important for companies to (gradually) connect suppliers to their own system or platform. The shipment status information (time stamp of the scans in the distribution centre/GPS data) needs to be sent by the transport service providers and received in real time. To do this, they also have to send the data via an interface.
This is why it makes sense for companies to define an IT architecture from the get-go, taking into account internal and external requirements, in order to set establish their own guidelines and specifications.
Data analytics
Now it is a matter of making the aggregated data usable for the company and analysing it. In an initial step, the data is presented in a dashboard in a way that is clear and specific to both the users and the requirements at hand. This makes the data transparent across the entire supply chain and makes it possible to detect anomalies early on.
Deviations from the plan can be visually highlighted in a dashboard and configured to include an alert that is sent to the appropriate entity, for example the customer service department. This makes it possible for customer service to transition from being reactive to being proactive. By integrating predictive analytics, patterns in historical data can be identified in order to predict future developments. This helps with things such as optimising purchasing and/or procurement forecasts. There are a multitude of other ways to work with the data. Which analysis tools make sense to use depends on the exact requirements.
Making a decision
It gets exciting when integrated trade-off analysers (based on prescriptive analytics) show various alternative courses of action with their cost and delivery time components. If, for example, it turns out that a primary product for the next calendar week’s scheduled production run will not arrive at the factory on time, the following options are available:
- Check stocks from other locations and determine their transport times and costs.
- Check whether the required product can be obtained from another supplier, including prices and changes in the production plan.
A sensible decision can be made based on this comprehensive cost and time analysis. In doing so, the focus is shifted from the linear supply chain to the entire supply network in order to exploit every potential and still meet critical customer orders.
These dynamic decision support systems are currently not yet included in the standard control tower. However, with the collaboration of data analytics experts, appropriate solutions can be set up according to the company’s needs.
Measures
Similar to autonomous driving, the overall responsibility is not completely relinquished. A person can decide on the next course of action to take based on the compilation of all the alternatives, including the recommendation. Having all the information available means countermeasures can be taken much more quickly. And if an unexpected situation occurs, a recalculation is performed immediately as opposed to waiting for fixed monthly/weekly planning cycles as used to be the case.
The system stores the adopted measure so that it can factor it in for future situations and continue learning, which falls within the domain of cognitive analytics. As soon as the decision for an alternative course of action has been made, the updated information should be automatically forwarded to the connected peripheral systems so that the relevant teams (internal and external) are informed about the changed situation and can take action.
Conclusion
In a self-driving supply chain, production and distribution automatically adapt to changing customer demands. In another scenario, having real-time information available makes it possible to take countermeasures to avoid production downtimes even in the event of late deliveries.
This concept, known as the self-driving supply chain, is an approach to consistently transporting goods in the best way possible in the future (from numerous suppliers to various production locations, to numerous customer addresses). That being said, is not just about speed, it is also about steady product availability, and transparency also makes it possible to consolidate transports for sustainability purposes.
Achieving this forward-looking goal requires close collaboration between experts from specialist departments such as purchasing, logistics, production planning and sales planning and IT experts from the enterprise architecture management and data analytics departments.
Anyone who would like to exchange ideas on this topic or knows a customer with a need is welcome to contact me: Nadine.Lamprecht@adesso.de
You can find out more about our services for companies in the field of mechanical and plant engineering on our website.
You can find more exciting topics from the adesso world in our blog posts published so far.
Glossary
- Predictive monitoring/analytics uses historical data to predict future events.
- Machine learning is an artificial system that is able to learn from examples and generalise them after completing its learning phase. To do this, machine learning algorithms construct a statistical model based on training data and test it against test data. This means that examples are not simply memorised, but rather patterns and regularities in the training data are identified.
- Robotic process automation is an approach to process automation in which what are known as software robots learn repetitive, manual, time-consuming or error-prone operations and perform them via an automated process.
- Prescriptive analytics is a form of business analysis that suggests possible decisions on how to exploit a future opportunity or mitigate a future risk and shows the effects of each possible decision.
- Cognitive analytics refers to tasks that could previously only be performed by people. Cognitive skills include things such as knowledge recall, analysis, comprehension, synthesis and evaluation (see Bloom’s Taxonomy). Modern AI technologies enable automated support for cognitive analytics.