27. January 2022 By Tim Strohschneider
Making relevant information usable with the help of modern artificial intelligence Text extraction use case
General consideration of the use of artificial intelligence (AI) in the banking world
In our AI study (available in Geman), one opinion is clear: those who invest in AI over the next five years will gain a competitive advantage. Therefore, this is relevant for all our customers.
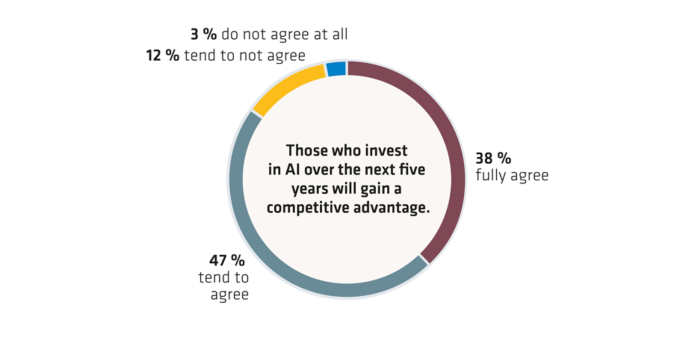
To break it down, content recognition is the most relevant aspect when using AI in the banking sector. Previous approaches to processing structured and unstructured information in the form of documents demanded a high degree of manual effort. Scanning routes are often monitored by humans and incoming customer communications are manually evaluated and processed.
Using this information manually is not only slow, but also the source of avoidable errors. A simple typo in the address can bring future customer communication to a standstill. This process can be significantly optimised with the help of text extraction. By using modern machine learning algorithms, we reduce the amount of manual effort needed, increase the quality of information processing and significantly increase the speed at which the process runs. A machine can be used in a scalable manner and thus adapt dynamically to the volume.
Support in the form of text extraction
In customer situations, we sketch out the current workflow in the Interaction Room: where do customer documents arrive, how are they processed, what is the output information and which format is it in. Once the procedure has been clarified, a target vision is defined. This means a picture of what the future process could look like, that is, after they have been optimised with the help of the possibilities offered by artificial intelligence. In a proof-of-concept (PoC), we build a neural network (usually SpaCy or Flair) for text extraction. This recognises entities (specific terms) and intents (the concern) in texts. This includes, for example, entities such as people, organisations, cities, products, addresses and much more.
Following this logic, the German word ‘Müller’, for example, can have many meanings in a text. The core competence of text extraction is to resolve ambiguities of names and words by linking them to the appropriate entities and concepts of a knowledge base. And what does that mean? Depending on the context, the word ‘Müller’ can refer to the profession Müller (‘miller’ in English), the health and beauty retailer chain Müller (similar to ‘Boots’ or ‘Superdrug’), German international footballer Thomas Müller and so on. Using text extraction means our solution easily finds out which detailed information the transmitted data or texts contain. The AI text extraction element assigns names and words their true meanings by comparing them to the knowledge base that been adapted to the company. In the same way, it is possible to group entities with synonyms. For instance, terms such as ‘Audi’, ‘BMW’ and ‘VW’ can all be recognised as synonyms of ‘car’ and assigned accordingly.
The use of text extraction approaches can therefore be applied in a number of ways and can turn unstructured documents into structured information. With the help of other technologies such as OCR, handwritten documents can also be digitised, processed and transferred to conversion systems.
Text extraction – reducing manual efforts in document management
Together with our customers, we implement a solution based on modern approaches that reduces or replaces manual processing steps. This then provides a significantly higher quality, faster and more reliably than a human can. At the same time, the solution is transparent, customisable and expandable. In this way, large, unstructured data sets can be resolved and made processable.
You will find more exciting topics from the adesso world in our latest blog posts.